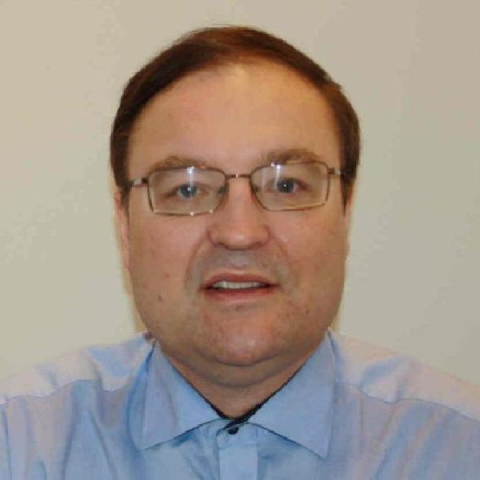
Enabling technologies for predictive digital twins
12.12.2023 at 13h30
ABSTRACT
We adopt the following definition of a Digital Twin [1]: A digital twin is defined as a virtual representation of a physical asset, or a process enabled through data and simulators for real-time prediction, optimization, monitoring, control, and decision-making.
To enable predictive twins, we utilize Hybrid Analysis and Modelling (HAM) [2-4] that combines classical Physic-Based Methods (PBM) accelerated by means of Reduced Order Modelling (ROM) [5-7] together with Data-Driven Methods (DDM) based on sensor measurement analysed by use of Machine Learning (ML).
We will in this talk present different ROM and HAM techniques applicable for structural mechanics and high Reynolds number fluid flow relevant for the maritime sector and renewable energy.
REFERENCES
A. Rasheed, O. San, T. Kvamsdal. Digital Twin: Values, Challenges and Enablers from a Modeling Perspective. IEEE Access, 8:21980--22012, 2020, 2020.
O. San, A. Rasheed, T. Kvamsdal. Hybrid analysis and modeling, eclecticism, and multifidelity computing toward digital twin revolution. GAMM‐Mitteilungen, e202100007. 2021.
S. Pawar, O. San, B. Aksoylu, A. Rasheed, T. Kvamsdal. Physics guided machine learning using simplified theories. Physics of Fluids, 33, 011701, 2021.
S. S. Blakseth, A. Rasheed, T. Kvamsdal, O. San. Deep neural network enabled corrective source term approach to hybrid analysis and modelling. Neural Networks, 146, 181-199, 2022.
E. Fonn, H. van Brummelen, T. Kvamsdal, and A. Rasheed. Fast divergence-conforming reduced basis methods for steady Navier-Stokes flow. Computer Methods in Applied Mechanics and Engineering, 346:486-512, 2019.
V. Tsiolakis, T. Kvamsdal, A. Rasheed, E. Fonn and H. van Brummelen. Reduced order models for finite-volume simulations of turbulent flow around wind-turbine blades. Journal of Physics: Conference Series, Volume 2018, 012042, 2021.
E. Fonn, H. van Brummelen, J. Lohne Eftang, T. Rusten, K. A. Johannessen,
T. Kvamsdald, A. Rasheed. Least-squares projected models for non-intrusive affinization of Reduced Basis Methods. Computer Methods in Applied Mechanics and Engineering, In review 2023.