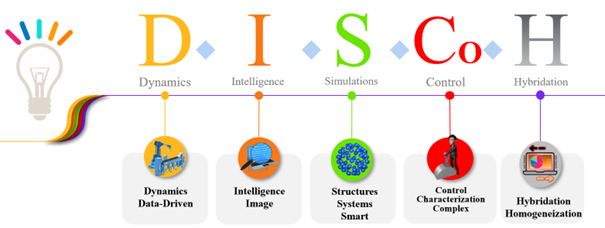
The DISCOH research group is one of the four academic research groups composing the PIMM laboratory. DISCOH members are located in the Arts & Métiers Campus in the center of Paris (here). Members of the team belong to the Ecole Nationale Supérieure des Arts et Métiers (ENSAM) or the Centre National de la Recherche Scientifique (CNRS).
In addition to its academic activities, the DISCOH research group has close relationships with industrial partners. The team is hosting SDT, a company specialized in experimental modal analysis and finite element modeling, a part-time associate professor funded by SAFRAN, and is leading several collaborative research chairs (ESI, SKF, RTE, ESTP, …).
Please follow the links below to know more about our research activities, who we are, the tools we developed, and some of our current projects.
- Research activities overview
- Research axis
- Staff members
- Available in-house tools
- Some highlights
- Academic and industrial collaborations
1) Research activities overview
a. Many research objects
What sets the DISCOH team apart is the rich application context in which it thrives. First and foremost, a wide range of input data relating to the dynamics of structures and processes are at the heart of its activities: images and videos at a few Hz, vibratory signals at a few kHz, or signals from guided waves (a few 100 kHz) or ultrasonic scans (a few MHz). The team's research also covers a wide range of application structures, mainly in the aerospace, automotive and rail industries. Finally, complex manufacturing processes are also part of the team's focus: historically machining, and more recently the manufacturing of composite materials (Resin Transfer Molding [RTM], Vacuum Assisted Resin Injection [VARI], ...) or 3D printing (either metallic or polymer).
b. A common scientific problematic
The DISCOH team is built up around a common scientific problematic which is:
“How can we make complex structures and processes smart via data- and physics-based models with both a controlled error and complexity?” |
The team is organized around four axes described in the following paragraphs: the first two axes focus on the problem of modeling, and the last two on adding smartness to structures and processes. All the team's axes are linked to numerical and experimental activities and are not independent of each other.
- Axis #1: Model reduction
- Axis #2: Physically Informed Machine Learning
- Axis #3: Smart Multifunctional Structures
- Axis #4: Smart Processes
By seeking to make complex structures and processes intelligent via data- and physics-based models, with controlled error and complexity, the DYSCO team is part of a dynamic that will ultimately have a potential ecological and environmental impact. The methodologies we are developing will enable us to move towards a more frugal approach to modeling, and to optimize the performances of structures and processes in terms of energy consumption, maintenance, and use of raw materials through the use of these new models.
2) Research axis
a. Axis #1: Model reduction
The DYSCO team's first axis of research is dedicated to model reduction. To be effective and efficient in complex industrial cases, numerical models must be predictive, with controlled error, and enable computations to be carried out in an acceptable time. The notion of "numerical model" covers both signal and system models, and ranges from purely data-based models to physical models such as finite element models. The scientific challenges addressed by the team in this theme are linked to the management of large parametric search spaces, the organization and exploration of large databases, the fusion of data from various sources, and the choice of models while controlling the induced error. Among the original approaches developed by the team in this area are the SDTools and nessy2m software packages, developed in-house, which enable non-linear simulations to be carried out on complex geometries, and Proper Generalized Decomposition (PGD), which enables hybrid reduced models to be built by combining physical knowledge and available data. The workflow allowing to build a reduced order model is depicted below.
![]() | ![]() |
Workflow of the parametric reduced order model builder | Structure-preserving matrix interpolation: in parametric settings, multiple reduced matrices are computed at user-selected parameter values. Each local matrix is only accurate in the neighborhood of its underlying training point. Interpolation techniques in matrix manifolds are used to output new reduced matrices for new parameter values. |
Parametric crash model, involving fast dynamics, buckling, contact and inelastic behavior (plasticity, damage and fracture). Depending on the thicknesses of four structural components the deformation can be evaluated and from it the quantities of interest: weight and intrusion. | Wind Map at Marina Bay district (Singapore): the wind velolicity at each point in the district is computed for any macroscopic wind intensity and direction provided by the wheater forecast or collected data (unperturbed by the district) – CNRS@CREATE courtesy, DesCartes programme |
![]() Reduction of piecewise periodic media using sparse sampling. Application to rail track transitions. Pinault, H., Arlaud, E., & Balmes, E. (2020). “A general superelement generation strategy for piecewise periodic media”. Journal of Sound and Vibration, 469. | ![]()
|
Key bibliographic references:
- “Regularized regressions for parametric models based on separated representations.” A. Sancarlos, V. Champaney, E. Cueto, F. Chinesta. Advanced Modeling and Simulation in Engineering Sciences, 10, Article number: 4 (2023)
- “Model Order Reduction.” F. Chinesta, A. Huerta, G. Rozza et K. Willcox. Encyclopedia of Computational Mechanics, Second Edition, 2015.
b. Axis #2: Physically Informed Machine Learning
The team's second axis of research focuses on physics-driven artificial intelligence. The tools associated with artificial intelligence (AI) are very powerful for making accurate and rapid predictions on cases for which a lot of training data is available. However, as soon as the study case changes slightly, these tools will not be able to adapt to the new practical case they are confronted with. On the other hand, it is very difficult to physically interpret AI models or, conversely, to infuse physical knowledge into an AI model. The key issues addressed by this area are therefore the search for methodologies enabling AI model architectures to be adapted to include physics or minimizing the need for training data by using the physical knowledge available a priori ("low data" approach). The team stands out here for its work on hybrid twins and the performance evaluation associated with these physics-informed AI models.
Analytical model | Exact solution (not real-time) | Physics informed solution (real-time) |
SKF: Active magnetic contactless bearings present an appealing alternative to classical bearings. However, the development of contactless bearings is impeded by the high nonlinearities inherent in the process and the need to accurately predict the bearing's behavior and levitation force within a fraction of a millisecond. This study implemented a machine learning algorithm informed by an analytical bearing model (left). The physics informed machine learning algorithm corrects the existing model in real-time without increasing computational cost (right). The correction model demonstrates high-accuracy predictions of the levitation force in comparison with the exact solution (center), even when tested on sets that were not previously encountered during the training process. |
![]() | ![]() |
Exact solution (not real-time) | Physics informed solution (real-time) |
Permafrost: The permafrost is a layer of earth that remains permanently frozen. However, this layer is currently thawing, resulting in earth cracks and structural collapses, happening actively throughout the planet. The objective of this project is to use existing permafrost measurements to forecast its future response amidst the climate changes we are experiencing. To achieve this goal, a novel machine learning algorithm has been developed, informed with physical inputs coming from OpenFoam Multiphysics simulations of the permafrost, aimed at enhancing predictions regarding permafrost behavior. Testing is performed over several months' unseen during the training of the physics informed machine learning algorithm. The predictions made by the developed model demonstrate a significant improvement in its predictive capabilities when compared to available simulations. |
Key bibliographic references:
- “Virtual, Digital and Hybrid Twins. A new paradigm in data-based engineering and engineered data.” F. Chinesta, E. Cueto, E. Abisset, J.L. Duval, F. El Khaldi. Archives of Computational Methods in Engineering, 27, 105–134, 2020.
- “Empowering Engineering with Data, Machine Learning and Artificial Intelligence: A Short Introductive Review.” F. Chinesta, E. Cueto. Advanced Modeling and Simulation in Engineering Sciences.
c. Axis #3: Smart Multifunctional Structures
The third axis of research is dedicated to the study of multifunctional structures. This area is motivated by the fact that structures designed today no longer have a purely "mechanical" role but include additional functions such as vibration control or autonomous monitoring of their state of health, with the aim of limiting their maintenance costs and carbon footprint. In this area, the team's main challenges are related to the choice of innovative active materials and their optimal deployment on these structures, the management of the operational context in which these structures operate, and the design of robust and adaptive algorithms to achieve the desired functions and new ones. The team's originality here lies in its ability to address this axis from both a numerical and an experimental point of view. What's more, with the PIMM's Polymers and Composites (P&C) team capable of manufacturing innovative active materials, the PIMM provides an ideal environment, rare in France, for this line of research.
![]() | ![]() | |
Prototype of a car door where the original electrodynamic loudspeaker has been replaced by piezoelectrical transducer directly bonded to the car door panels. See the articles A & B by N. Benbara for more information. | REMAP experimental fatigue test campaign on composite aeronautic structures. Data available in open access. | PVDF soft actuator electromechanical characterization. From the Ph.D thesis of S. Zanchi. |
Key bibliographic references:
- Rébillat, M. & Mechbal, N. “Damage localization in geometrically complex aeronautic structures using canonical polyadic decomposition of Lamb wave difference signal tensors” Structural Health Monitoring, 2020, 19, 305-321
- Benbara, N.; Rébillat, M. & Mechbal, N. “Bending waves focusing in arbitrary shaped plate-like structures: Application to spatial audio in cars” Journal of Sound and Vibration, 2020, 487
d. Axis #4: Smart Processes
The team's fourth axis is to move towards intelligent manufacturing processes. Standard manufacturing processes (machining, composite materials manufacturing, 3D printing) usually operate in an open-loop mode, in the sense that there are no real-time quality measurements on the structures being manufactured, enabling certain process parameters to be corrected on-line, to limit scrap, for example. The scientific hurdles to be overcome in order to achieve this objective include the fact that processes are non-linear dynamic systems that do not evolve over time, the fact that it is often difficult in practice to instrument a manufacturing process, and finally the fact that instabilities can occur in the process during closed-loop operation. The team's main distinguishing feature here is its long-standing expertise in machining simulation and instrumentation. Here again, proximity to the LASER, COMET and P&C teams creates a highly favorable environment for this theme, and it is in this context that work on 3D printing, VARI and RTM processes has been initiated more recently.
Hybrid twin model of the RTM process showing the resin flow front propagation evolution with time. For more information see the article of S. Iturra. | Automated fiber placement simulation |
Topological optimization of a 3D printed structure |
Key bibliographic references:
- Rodriguez, S., Monteiro, E., Mechbal, N., Rebillat, M., & Chinesta, F. (2023). “Hybrid twin of RTM process at the scarce data limit.” International Journal of Material Forming, 16(4), 40.
- Toubhans, B., Lorong, P., Viprey, F., Fromentin, G., & Karaouni, H. (2021). “A versatile approach, considering tool wear, to simulate undercut error when turning thin-walled workpieces.” The International Journal of Advanced Manufacturing Technology, 115(5-6), 1919-1929.
3) Staff members
The DISCOH team is built up around 5 full professor, 2 partial time full professor, 6 associate professor and 2 research engineers. We usually host 10 to 15 Ph.D. students and around 5 post-doctoral researchers. This multi-disciplinary team focuses on the dynamics of structures and processes, with skills in numerical mechanics, experimental dynamics, signal processing and the control of smart structures & processes.
![]() | ![]() | ![]() | ![]() | ![]() |
![]() | ![]() | ![]() | ![]() | ![]() |
![]() | ![]() | ![]() | ![]() | ![]() |
4) Available in-house tools
The team has forged its own tools to address the targeted challenges. These tools are either numerical software or experimental facilities.
a. SDTools
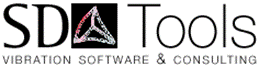
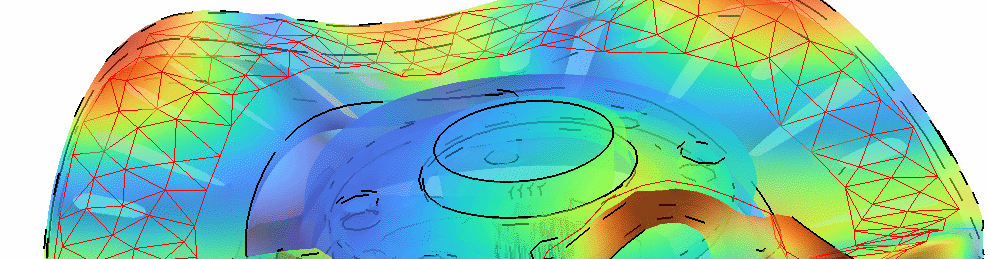
SDTools specializes in providing open and extensible MATLAB® based solutions in experimental modal analysis and finite element modeling for vibration problems. It enhances MATLAB core capabilities in controls and signal processing through extensions linked to general 3-D finite element modeling, experimental modal analysis, or test &analysis correlation. SDTools is developed and maintained by E. Balmes.
b. Nessi2M
The nessy2m program is dedicated to the simulation of machining operations: milling, boring and turning. Its main goal is to predict the defects on the geometry of machined surface: form, waviness and partly roughness. This software is developed and maintained by P. Lorong et A. Illoul.
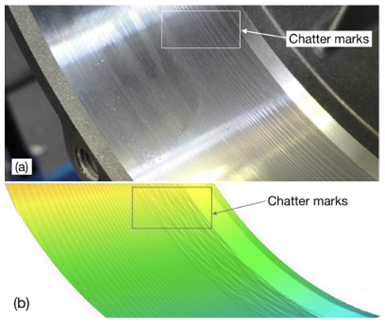
c. SHM@PIMM
SHM@PIMM is both an experimental platform and a signal processing software dedicated to the acquisition and analysis Lamb wave signals used for the structural health monitoring of thin structures. The experimental platform allows to acquire ultrasonic data in the range of 100~kHz to 300~kHz and the associated MATLAB toolbox to process those data for damage monitoring purposes. The software is developed and maintained by M. Rébillat.
![]() |
5) Academic and industrial collaborations
a. Invited professors
![]() Trond KVAMSDAL | ![]() Pedro PONTE CASTANEDA | ![]() Samuel DA SILVA |
![]() Charbel FARHAT | ![]() Izhak BUCHER |
b. Industrial research chairs
![]() | Digital twins of construction and infrastructure in their environment
|
![]() | ESI Group Chair “CREATE ID”
|
![]() | SKF Chair
|
![]() | RTE Chair
|
![]() | KEYSIGHT Chair
|
6) Highlights
a. Time integration strategies and numerical experimental designs
Recent projects carried out within the team have led to gains of two orders of magnitude in time integration strategies, leading to a complete review of strategies for the realization and analysis of nonlinear vibration experimental designs in calculation and testing. Applications include squeal, high-strain damping and wheel-rail contact.
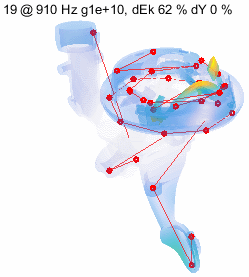
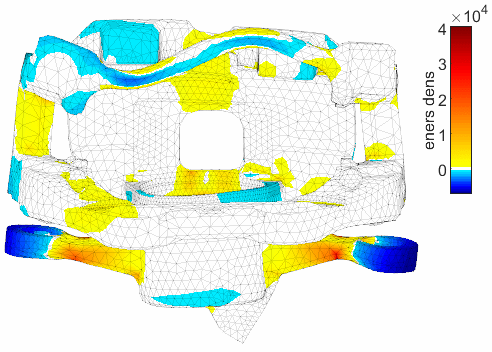
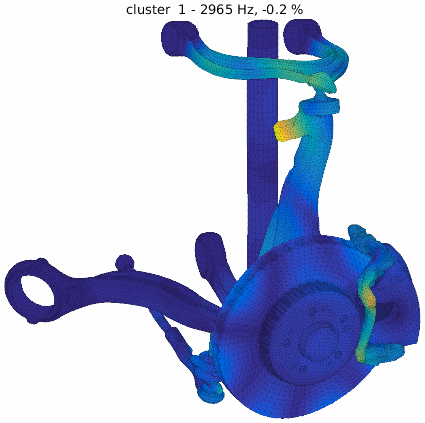
Parametric superelements are used for expansion (the hybrid combination of test and reduced FEM for the estimation of states and parameters) and extensive parametric studies. Martin, G., Vermot Des Roches, G., Balmes, E., & Chancelier, T. (2019). MDRE: an efficient expansion tool to perform model updating from squeal measurements.
b. Model reduction, data-driven models and hybridization
In the context of model order reduction, our proposals concerned three kind of separated representations:
- Space-time enabling non-incremental procedures, also formulated in alternative spaces (Fourier, …)
- Space dimensions, enabling the solution of multi-physic problems defined in degenerated domains, like plate, shell or beam-like geometries
- Parameters as extra-coordinates, enabling the offline calculation of parametric solutions that then, can be particularized in real-time.
When the usual separated representation finds difficulties to address extremely nonlinear models, the so-called non-intrusive model order reduction based on the construction of efficient surrogates able to operate in high-dimensional spaces becomes a valuable alternative.
To illustrate this point, we can mention two items:
- F. Chinesta's leadership of the DESCARTES (Decision-making in Critical Urban Systems) program.
- The following publication defining the notion of hybrid twin: Chinesta, F. et al. "Virtual, Digital and Hybrid Twins: A New Paradigm in Data-Based Engineering and Engineered Data" Archives of Computational Methods in Engineering, 27, 105-134, 2020.
c. Multifunctional structures from manufacturing to recycling
The DISCOH team is responsible for coordinating the H2020 MORPHO project (by N. Mechbal), which aims to demonstrate the industrial interest of multifunctional structures by considering their entire life cycle, i.e. from manufacturing to recycling.
MORPHO is an industrial research project, financed by the Horizon 2020 program with a budget of €5 million, whose ambition is to accompany the development of smart aeronautical parts namely engine fan blades, to endow them with cognitive capabilities, and to optimize their manufacturing and their life cycle management.
MORPHO "Manufacturing, Overhaul, Repair for Prognosis Health Overreach" aims to, design, develop, test and validate an innovative, robust, and environmentally friendly industrial process to manufacture, monitor, and recycle a new generation of intelligent, multifunctional, multi-material parts such as engine fan blades. This process will rely on embedded sensors, data-driven hybrid twins, and machine learning algorithms to track the entire life cycle of the targeted aeronautical parts in real-time.
The final goal of MORPHO is to contribute to developing standards that will enable a reliable, sustainable, agile, and cost-competitive industrialization of smart structures for aeronautics.
d. Dynamic simulation of complex non-linear systems
This theme builds on actions linked to themes #1 and #2, and in particular the machining process, for which the nessy2m software capitalizes on advances. The software, which is moving towards open source distribution (under LGPL license), is beginning to spread in the industrial world, and is used by Framatome and Safran Tech in particular. The following video illustrates a machining example in which the part and tool are subject to considerable vibration.